Bird migration analysis example¶
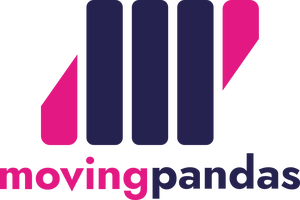
This tutorial uses data published on Movebank, specifically: Navigation experiments in lesser black-backed gulls (data from Wikelski et al. 2015)-gps.csv
This tutorial covers:
- Trajectory data preprocessing
- Loading movement data from common geospatial file formats
- Exploring spatial & non-spatial data distributions
- Converting GeoDataFrames into Trajectories describing continuous tracks of moving objects
- Trajectory data analysis
- Investigating individual trajectories
- Comparing different years
- Investigating trajectories of multiple individuals
import numpy as np
import pandas as pd
import geopandas as gpd
import movingpandas as mpd
import shapely as shp
import hvplot.pandas
import matplotlib.pyplot as plt
from geopandas import GeoDataFrame, read_file
from shapely.geometry import Point, LineString, Polygon
from datetime import datetime, timedelta
from holoviews import opts, dim
from os.path import exists
from urllib.request import urlretrieve
import warnings
warnings.filterwarnings("ignore")
plot_defaults = {"linewidth": 5, "capstyle": "round", "figsize": (9, 3), "legend": True}
opts.defaults(
opts.Overlay(active_tools=["wheel_zoom"], frame_width=300, frame_height=500)
)
hvplot_defaults = {"tiles": None, "cmap": "Viridis", "colorbar": True}
mpd.show_versions()
MovingPandas 0.20.0 SYSTEM INFO ----------- python : 3.10.15 | packaged by conda-forge | (main, Oct 16 2024, 01:15:49) [MSC v.1941 64 bit (AMD64)] executable : c:\Users\Agarkovam\AppData\Local\miniforge3\envs\mpd-ex\python.exe machine : Windows-10-10.0.19045-SP0 GEOS, GDAL, PROJ INFO --------------------- GEOS : None GEOS lib : None GDAL : None GDAL data dir: None PROJ : 9.5.0 PROJ data dir: C:\Users\Agarkovam\AppData\Local\miniforge3\envs\mpd-ex\Library\share\proj PYTHON DEPENDENCIES ------------------- geopandas : 1.0.1 pandas : 2.2.3 fiona : None numpy : 1.23.1 shapely : 2.0.6 pyproj : 3.7.0 matplotlib : 3.9.2 mapclassify: 2.8.1 geopy : 2.4.1 holoviews : 1.20.0 hvplot : 0.11.1 geoviews : 1.13.0 stonesoup : 1.4
Loading the bird movement data¶
%%time
df = read_file("../data/gulls.gpkg")
print(f"Finished reading {len(df)}")
Finished reading 89867 CPU times: total: 1.3 s Wall time: 1.32 s
This is what the data looks like:
df.head()
event-id | visible | timestamp | location-long | location-lat | sensor-type | individual-taxon-canonical-name | tag-local-identifier | individual-local-identifier | study-name | geometry | |
---|---|---|---|---|---|---|---|---|---|---|---|
0 | 1082620685 | true | 2009-05-27 14:00:00.000 | 24.58617 | 61.24783 | gps | Larus fuscus | 91732 | 91732A | Navigation experiments in lesser black-backed ... | POINT (24.58617 61.24783) |
1 | 1082620686 | true | 2009-05-27 20:00:00.000 | 24.58217 | 61.23267 | gps | Larus fuscus | 91732 | 91732A | Navigation experiments in lesser black-backed ... | POINT (24.58217 61.23267) |
2 | 1082620687 | true | 2009-05-28 05:00:00.000 | 24.53133 | 61.18833 | gps | Larus fuscus | 91732 | 91732A | Navigation experiments in lesser black-backed ... | POINT (24.53133 61.18833) |
3 | 1082620688 | true | 2009-05-28 08:00:00.000 | 24.58200 | 61.23283 | gps | Larus fuscus | 91732 | 91732A | Navigation experiments in lesser black-backed ... | POINT (24.582 61.23283) |
4 | 1082620689 | true | 2009-05-28 14:00:00.000 | 24.58250 | 61.23267 | gps | Larus fuscus | 91732 | 91732A | Navigation experiments in lesser black-backed ... | POINT (24.5825 61.23267) |
df.plot()
<Axes: >
Let's see how many individuals we have in the dataset:
df["individual-local-identifier"].unique()
array(['91732A', '91733A', '91734A', '91735A', '91737A', '91738A', '91739A', '91740A', '91741A', '91742A', '91743A', '91744A', '91745A', '91746A', '91747A', '91748A', '91749A', '91750A', '91751A', '91752A', '91754A', '91755A', '91756A', '91758A', '91759A', '91761A', '91762A', '91763A', '91764A', '91765A', '91766A', '91767A', '91769A', '91771A', '91774A', '91775A', '91776A', '91777A', '91778A', '91779A', '91780A', '91781A', '91782A', '91783A', '91785A', '91786A', '91787A', '91788A', '91789A', '91794A', '91795A', '91797A', '91798A', '91799A', '91800A', '91802A', '91803A', '91807A', '91809A', '91810A', '91811A', '91812A', '91813A', '91814A', '91815A', '91816A', '91819A', '91821A', '91823A', '91824A', '91825A', '91826A', '91827A', '91828A', '91829A', '91830A', '91831A', '91832A', '91835A', '91836A', '91837A', '91838A', '91839A', '91843A', '91845A', '91846A', '91848A', '91849A', '91852A', '91854A', '91858A', '91861A', '91862A', '91864A', '91865A', '91866A', '91870A', '91871A', '91872A', '91875A', '91876A', '91877A', '91878A', '91880A', '91881A', '91884A', '91885A', '91893A', '91894A', '91897A', '91900A', '91901A', '91903A', '91907A', '91908A', '91910A', '91911A', '91913A', '91916A', '91918A', '91919A', '91920A', '91921A', '91924A', '91929A', '91930A'], dtype=object)
The records per individual are not evenly distributed:
df["individual-local-identifier"].value_counts().plot(kind="bar", figsize=(17, 3))
<Axes: xlabel='individual-local-identifier'>
Finally, let's create trajectories:
tc = mpd.TrajectoryCollection(
df, "individual-local-identifier", t="timestamp", min_length=100
)
tc
TrajectoryCollection with 125 trajectories
And let's generalize them to speed up the following analyses:
tc = mpd.MinTimeDeltaGeneralizer(tc).generalize(tolerance=timedelta(days=1))
Investigating individual trajectories¶
Let's pick out a specific individual. For example, '91916A' is the individual with most records in our dataset:
filtered = tc.filter("individual-local-identifier", "91916A")
my_traj = filtered.trajectories[0].copy()
my_traj.df.head()
event-id | visible | location-long | location-lat | sensor-type | individual-taxon-canonical-name | tag-local-identifier | individual-local-identifier | study-name | geometry | |
---|---|---|---|---|---|---|---|---|---|---|
timestamp | ||||||||||
2009-08-15 15:00:00 | 1082625177 | true | 7.91500 | 54.18533 | gps | Larus fuscus | 91916 | 91916A | Navigation experiments in lesser black-backed ... | POINT (7.915 54.18533) |
2009-08-16 15:00:00 | 1082625181 | true | 9.44183 | 54.87233 | gps | Larus fuscus | 91916 | 91916A | Navigation experiments in lesser black-backed ... | POINT (9.44183 54.87233) |
2009-08-17 15:00:00 | 1082625185 | true | 9.44250 | 54.87283 | gps | Larus fuscus | 91916 | 91916A | Navigation experiments in lesser black-backed ... | POINT (9.4425 54.87283) |
2009-08-18 15:00:00 | 1082625189 | true | 9.41167 | 54.85550 | gps | Larus fuscus | 91916 | 91916A | Navigation experiments in lesser black-backed ... | POINT (9.41167 54.8555) |
2009-08-19 15:00:00 | 1082625193 | true | 9.39583 | 54.88000 | gps | Larus fuscus | 91916 | 91916A | Navigation experiments in lesser black-backed ... | POINT (9.39583 54.88) |
my_traj.hvplot(title=f"Movement of {my_traj.id}", line_width=2)
This individual has been travelling back and forth for quite a few years!
One way to take a closer look at this individual's travels is to split the overall track into yearly trips:
trips_by_year = mpd.TemporalSplitter(filtered).split(mode="year")
trips_by_year.to_traj_gdf()
individual-local-identifier | start_t | end_t | geometry | length | direction | |
---|---|---|---|---|---|---|
0 | 91916A_2009-12-31 00:00:00 | 2009-08-15 15:00:00 | 2009-12-31 19:00:00 | LINESTRING (7.915 54.18533, 9.44183 54.87233, ... | 5.352375e+06 | 131.939002 |
1 | 91916A_2010-12-31 00:00:00 | 2010-01-01 19:00:00 | 2010-12-31 07:00:00 | LINESTRING (39.18417 21.17583, 39.18833 21.170... | 1.232428e+07 | 281.047091 |
2 | 91916A_2011-12-31 00:00:00 | 2011-01-01 07:00:00 | 2011-12-31 04:00:00 | LINESTRING (39.17 21.18017, 39.16883 21.15633,... | 1.441793e+07 | 189.238229 |
3 | 91916A_2012-12-31 00:00:00 | 2012-01-01 04:00:00 | 2012-12-31 19:00:00 | LINESTRING (39.16933 21.16667, 39.17567 21.142... | 1.324811e+07 | 62.132640 |
4 | 91916A_2013-12-31 00:00:00 | 2013-01-01 19:00:00 | 2013-12-31 13:00:00 | LINESTRING (39.18167 21.17333, 39.181 21.17317... | 1.293261e+07 | 273.165851 |
5 | 91916A_2014-12-31 00:00:00 | 2014-01-01 13:00:00 | 2014-12-31 19:00:00 | LINESTRING (39.17083 21.154, 39.171 21.1575, 3... | 1.300973e+07 | 33.742967 |
6 | 91916A_2015-12-31 00:00:00 | 2015-01-01 19:00:00 | 2015-08-27 09:00:00 | LINESTRING (39.18167 21.16967, 39.18233 21.169... | 6.551740e+06 | 343.887905 |
Now we can explore individual years:
one_year = trips_by_year.get_trajectory("91916A_2010-12-31 00:00:00")
one_year
Trajectory 91916A_2010-12-31 00:00:00 (2010-01-01 19:00:00 to 2010-12-31 07:00:00) | Size: 354 | Length: 12324280.3m Bounds: (18.53417, 21.047, 39.203, 61.543) LINESTRING (39.18417 21.17583, 39.18833 21.17083, 39.18767 21.172, 39.1865 21.17117, 39.18817 21.170
one_year.add_speed(units=("km", "h"))
Trajectory 91916A_2010-12-31 00:00:00 (2010-01-01 19:00:00 to 2010-12-31 07:00:00) | Size: 354 | Length: 12324280.3m Bounds: (18.53417, 21.047, 39.203, 61.543) LINESTRING (39.18417 21.17583, 39.18833 21.17083, 39.18767 21.172, 39.1865 21.17117, 39.18817 21.170
one_year.hvplot(
title=f"Movement speed of {one_year.id}",
line_width=5.0,
c="speed",
cmap="RdYlGn",
colorbar=True,
clim=(0, 20),
)
Let's see where this individual was on a specific day:
def plot_location_at_timestamp(traj, t, fig_size=250):
loc = GeoDataFrame([traj.get_row_at(t)])
return loc.hvplot(
title=str(t), geo=True, tiles="OSM", size=200, color="red"
) * traj.hvplot(
line_width=1.0, color="black", tiles=False, width=fig_size, height=fig_size
)
(
plot_location_at_timestamp(one_year, datetime(2010, 9, 1))
+ plot_location_at_timestamp(one_year, datetime(2010, 10, 1))
+ plot_location_at_timestamp(one_year, datetime(2010, 11, 1))
)
Of course, it might also be of interest to see the different locations on a certain day each year:
def plot_location_at_day_of_year(traj, month, day, ax=None):
ts = [datetime(year, month, day) for year in traj.df.index.year.unique()]
return plot_locations_at_timestamps(traj, ts, ax=ax)
def plot_locations_at_timestamps(traj, ts, ax=None):
loc = GeoDataFrame([traj.get_row_at(t) for t in ts])
loc["date_label"] = loc.index.strftime("%Y-%m-%d")
return loc.hvplot(
title=f"Movement of {traj.id}", c="date_label", size=200, geo=True, tiles="OSM"
) * traj.hvplot(line_width=1.0, color="black", geo=True, tiles=False)
plot_location_at_day_of_year(my_traj, month=10, day=1)
It's pretty clear that this individual does not follow the same schedule and route every year. However, it seems to always be heading to the same area Red Sea coast to spend the winter there.
Let's find its arrival times in this area:
area_of_interest = Polygon([(30, 25), (50, 25), (50, 15), (30, 15), (30, 25)])
plotted_area_of_interest = GeoDataFrame(
pd.DataFrame([{"geometry": area_of_interest, "id": 1}]), crs=4326
).hvplot(geo=True, color="yellow", alpha=0.5)
arrivals = [traj for traj in my_traj.clip(area_of_interest)]
print(f"Found {len(arrivals)} arrivals")
for traj in arrivals:
print(
f"Individual '{traj.df['individual-local-identifier'].iloc[0]}' arrived at {traj.get_start_time()}"
)
Found 6 arrivals Individual '91916A_2009-12-23 02:58:36.946571' arrived at 2009-12-23 02:58:36.946571 Individual '91916A_2010-10-30 20:55:36.697832' arrived at 2010-10-30 20:55:36.697832 Individual '91916A_2011-11-09 20:25:19.550486' arrived at 2011-11-09 20:25:19.550486 Individual '91916A_2012-10-14 11:25:28.063070' arrived at 2012-10-14 11:25:28.063070 Individual '91916A_2013-10-08 04:17:33.524488' arrived at 2013-10-08 04:17:33.524488 Individual '91916A_2014-10-28 19:05:32.941608' arrived at 2014-10-28 19:05:32.941608
(
plot_locations_at_timestamps(my_traj, [traj.get_start_time() for traj in arrivals])
* plotted_area_of_interest
)
Investigating trajectories of multiple individuals¶
Multiple individuals travel to this area every year. Let's have a closer look:
year_of_interest = 2010
trajs_in_aoi = tc.clip(area_of_interest)
relevant = [
traj
for traj in trajs_in_aoi
if traj.get_start_time().year <= year_of_interest
and traj.get_end_time().year >= year_of_interest
]
print("Found {} arrivals".format(len(relevant)))
Found 16 arrivals
for traj in relevant:
print(
"Individual '{}' arrived at {} (duration: {})".format(
traj.df["individual-local-identifier"].iloc[0],
traj.get_start_time().date(),
traj.get_end_time() - traj.get_start_time(),
)
)
Individual '91732A_2010-04-10 01:46:26.971138' arrived at 2010-04-10 (duration: 5 days, 21:27:11.670985) Individual '91737A_2009-12-08 19:48:30.526794' arrived at 2009-12-08 (duration: 140 days, 11:11:29.473206) Individual '91761A_2010-04-11 21:07:47.330927' arrived at 2010-04-11 (duration: 12 days, 6:10:03.857850) Individual '91761A_2010-10-04 21:51:17.376565' arrived at 2010-10-04 (duration: 6 days, 10:42:00.340093) Individual '91762A_2010-04-19 18:31:37.300934' arrived at 2010-04-19 (duration: 42 days, 1:28:22.699066) Individual '91771A_2009-12-23 11:54:28.946218' arrived at 2009-12-23 (duration: 66 days, 8:05:31.053782) Individual '91789A_2009-11-10 23:48:44.382330' arrived at 2009-11-10 (duration: 550 days, 20:39:18.769409) Individual '91824A_2010-05-06 01:06:06.590764' arrived at 2010-05-06 (duration: 12:53:53.409236) Individual '91832A_2010-04-21 04:03:50.548155' arrived at 2010-04-21 (duration: 3 days, 5:48:46.276706) Individual '91832A_2010-09-23 06:51:31.595353' arrived at 2010-09-23 (duration: 1 day, 1:40:25.175880) Individual '91837A_2010-05-04 02:28:18.858339' arrived at 2010-05-04 (duration: 1 day, 18:38:46.170554) Individual '91846A_2010-05-15 22:50:49.280981' arrived at 2010-05-15 (duration: 10 days, 2:50:28.505732) Individual '91862A_2010-01-06 04:21:17.332648' arrived at 2010-01-06 (duration: 248 days, 6:10:16.962620) Individual '91910A_2010-09-28 01:19:48.348387' arrived at 2010-09-28 (duration: 2 days, 20:34:31.117736) Individual '91916A_2009-12-23 02:58:36.946571' arrived at 2009-12-23 (duration: 115 days, 11:33:05.224061) Individual '91916A_2010-10-30 20:55:36.697832' arrived at 2010-10-30 (duration: 154 days, 14:44:36.105271)
Based on the duration of the individuals' trajectory segments within our area of interest, it looks like some individuals spend the winter here while others only pass through.
For example, Individual '91761A' passed through twice? What has it been up to?
my_traj = tc.get_trajectory("91761A")
segment = my_traj.get_segment_between(
datetime(year_of_interest, 1, 1), datetime(year_of_interest, 12, 31)
)
segment.hvplot(color="black", line_width=1.0) * plotted_area_of_interest
Turns out that this individual does not stay at the Red Sea but continues its journey into Africa.